- Subscribe to RSS Feed
- Mark as New
- Mark as Read
- Bookmark
- Subscribe
- Printer Friendly Page
- Report Inappropriate Content
Created on 09-15-2022 04:35 PM - edited 06-26-2023 05:07 AM
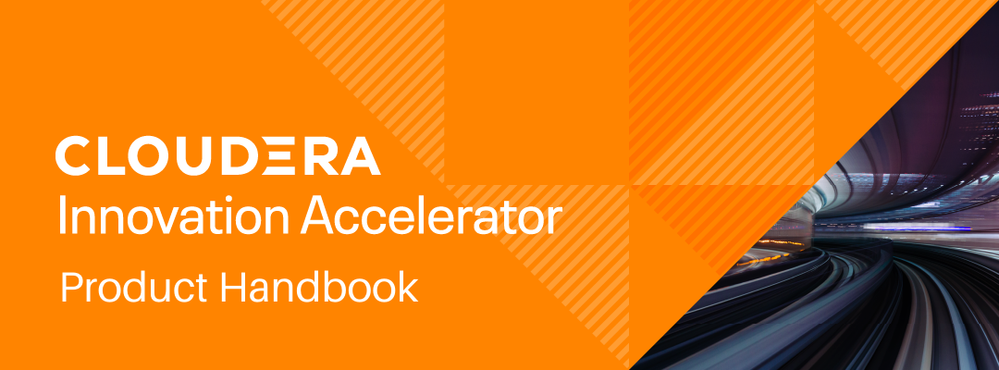
Overview
Cloudera has implemented dbt adapters for Hive, Spark (CDE and Livy), and Impala. In addition to providing the adapters, Cloudera is offering a turn-key solution to be able to manage the end-to-end software development life cycle (SDLC) of dbt models. This solution is available in all clouds as well as on-prem deployments of CDP. It is useful for customers who would not like to use dbt Cloud for security reasons or the lack of availability of adapters in dbt cloud.
We have identified the following requirements for any solution that supports the end-to-end SDLC of data transformation pipelines using dbt.
- Have multiple environments
- Dev
- Stage/Test
- Prod
- Have a dev setup where different users can do the following in an isolated way:
- Make changes to models
- Test changes
- See logs of tests
- Update docs in the models and see docs
- Have a CI/CD pipeline to push committed changes in the git repo to stage/prod environments
- See logs in stage/prod of the dbt runs
- See dbt docs in stage/prod
- Orchestration: Ability to run dbt run regularly to update the models in the warehouse, or based on events(Kafka)
- Everything should be part of one application(tool) like CDP or CDSW
- Alerting and Monitoring, if there is a failure how IT team will know that
Any deployment for dbt should also satisfy the following
- Convenient for analysts - no terminal/shells/installing software on a laptop. Should be able to use a browser.
- Support isolation across different users using it in dev
- Support isolation between different environments (dev/stage/prod)
- Secure login - SAML
- Be able to upgrade the adapters or core seamlessly
- Vulnerability scans and fixing CVEs
- Able to add and remove users for dbt - Admin privilege
Cloudera Data Platform has a service, CDSW, which offers users the ability to build and manage all of their machine learning workloads. The same capabilities of CDSW can also be used to satisfy the requirements for the end-to-end SDLC of dbt models.
In this document, we will show how an admin can set up the different capabilities in CDSW like workspaces, projects, sessions, and runtime catalogs so that an analyst can work with their dbt models without having to worry about anything else.
First, we will show how an admin can set up
- CDSW runtime catalog within a workspace, with the Cloudera provided container with dbt-core and all adapters supported by Cloudera
- CDSW project for stage/prod (i.e., automated/non-development) environments. Analysts create their own projects for their development work.
- CDSW jobs to run the following commands in an automated way on a regular basis
- git clone
- dbt debug
- dbt run
- dbt doc generate
- CDSW apps to serve model documentation in stage/prod
Next, we will show how an analyst can build, test, and merge changes to dbt models by using
- CDSW project to work in isolation without being affected by other users
- CDSW user sessions - for interactive IDE of dbt models
- git to get the changes reviewed and pushed to production
Finally, we will show how by using CDSW all of the requirements for the end-to-end software development lifecycle of dbt models.
Administrator steps
Prerequisites
- A CDSW environment is required to deploy dbt with CDSW, refer Installing Cloudera Data Science Workbench on CDP to create an environment
- Administrator should have access to the CDP-Base Control Plane and admin permissions to CDSW
- Access to a git repository with basic dbt scaffolding (using proxies if needed). If such a repository does not exist, follow the steps in Getting started with dbt Core
- Access to custom runtime catalog (using proxies if needed)
- Machine user credentials - user/pass or Kerberos - for stage and production environments. See CDP machine user on creating machine users for hive/impala/spark.
Note: The document details a simple setup within CDSW where we will
|
Step 1. Create and enable a custom runtime in CDSW with dbt
In the workspace screen, click on “Runtime Catalog” to create a custom runtime with dbt.
Step 1.1. Create a new runtime environment
- Select the Runtime Catalog from the side menu, and click Add Runtime button:
- Use the following URL for Docker Image to Upload: http://public.ecr.aws/d7w2o6p0/dbt-cdsw:1.1.11 Click on Validate.
- When validation succeeds, click on “Add to Catalog”.
- The new runtime will show up in the list of runtimes
Step 1.2. Set runtime as default for all new sessions
- In the workspace’s side menu, select Site Administration and scroll down to the Engine Images section.
- Add a new Engine image by adding the following values
Field
Value
Description
Description
dbt-cdsw
Respository:Tag
Default
Enable
Make this runtime the default for all new sessions in this workspace
Step 2. Set up projects for stage and prod (automated) environments
Admins create projects for stage and prod (and other automated) environments. Analysts can create their own projects.
Creating a new project for stage/prod requires the following steps:
- Create a CDSW project
- Set up environment variables for credentials and scripts
Step 2.1. Create a CDSW project
- From the workspace screen, click on Add Project
- Fill out the basic information for the project
Field
Value
Notes
Project Name
prod-marketing
Name of the dbt project running in stage/prod
Project Description
Project Visibility
Private
Recommend private for prod and stage
Initial Setup
Blank
We will set up git repos separately via CDSW Jobs later in prod/stage.
- Click the Create Project button on the bottom right corner of the screen
Step 2.2. Set environment variables to be used in automation
To avoid checking profile parameters (users credentials) to git, the user SSH key can be configured for access to the git repo (How to work with GitHub repositories in CML/CDSW - Cloudera Community - 303205)
- Click Project Settings from the side menu on the project home page and click on Advanced tab
- Enter the environment variables. Click on
to add more environment variables.
Key
Value
Notes
DBT_GIT_REPO
Repository that has the dbt models and profiles.yml
DBT_IMPALA_HOST
DBT_IMPALA_HTTP_PATH
DBT_IMPALA_USER
DBT_IMPALA_PASSWORD
DBT_IMPALA_DBNAME
DBT_IMPALA_SCHEMA
DBT_SPARK_CDE_HOST
DBT_SPARK_CDE_AUTH_ENDPOINT
DBT_SPARK_CDE_PASSWORD
DBT_SPARK_CDE_USER
DBT_SPARK_CDE_SCHEMA
DBT_SPARK_LIVY_HOST
DBT_SPARK_LIVY_USER
DBT_SPARK_LIVY_PASSWORD
DBT_SPARK_LIVY_DBNAME
DBT_SPARK_LIVY_SCHEMA
DBT_HIVE_HOST
DBT_HIVE_HTTP_PATH
DBT_HIVE_USER
DBT_HIVE_PASSWORD
DBT_HIVE_SCHEMA
Adapter specific configs passed as environment variables
DBT_HOME
Path to home directory
Note:
There could be different environment variables that need to be set depending on the specific engine and access methods like Kerberos or LDAP. Refer to the engine-specific adapter documentation to get the full list of parameters in the credentials.
- Environment variables will look as shown below:
- Click the Submit button on the right side of the section
Note: dbt_impala_demo: |
Note: Environment variables are really flexible. You can use them for any field in the profiles.yml jaffle_shop: |
In Step 3.3. Setup dbt debug job, you will be able to test and make sure that the credentials provided to the warehouse are accurate.
Step 3. Create jobs and pipeline for stage/prod
CDSW jobs will be created for the following jobs to be run in order as a pipeline to be run on a regular basis whenever there is a change pushed to the dbt models repository.
- Get the scripts for the different jobs
- git clone/pull
- dbt debug
- dbt run
- dbt docs generate
All the scripts for the jobs are available in the custom runtime that is provided. These scripts rely on the project environment variables that have been created in the previous section.
Step 3.1 Authenticate
Before starting a session you may need to authenticate, the steps may vary based on the authentication mechanism.
- Go to User Settings from the left menu:
- If your instance uses the Kerberos mechanism, select Hadoop Authentication and fill in the Principal and Credentials and click Authenticate.
Step 3.2 Setup scripts location
Scripts are present under the /scripts folder as part of the dbt custom runtime. However, the CDSW jobs file interface only lists the files under the home directory (/home/cdsw).
Create a session with the custom runtime:
and from the terminal command line, copy the scripts to the home folder.
cp -r /scripts /home/cdsw/
Step 3.3 Setup git clone job
Create a new job for git clone and select the job script from the scripts folder updated in Step 3.2
Update the arguments and environment variables, and create the job.
Field Name | Value | Comment |
Name | job-git-clone | |
Script | scripts/job-git-clone.py | This is the script that would be executed in this job step. |
Arguments | /home/cdsw/dbt-impala-example/dbt_impala_demo | Path of the dbt project file, which is part of the repo. |
Editor | Workbench | |
Kernel | Python 3.9 | |
Edition | dbt cdsw custom runtime | |
Version | 1.1 | |
Runtime Image | Find most updated docker image here | |
Schedule | Recurring; Every hour | This can be configured as either Manual/Recurring or Dependent |
Use a cron expression | Check, 0**** | Default value |
Resource profile | 1vCPU/2GiB | |
Timeout In Minutes | - | Optional timeout for the job |
Environment Variables | These can be used to overwrite settings passed at project level (Section 3.2) | |
Job Report Recipients | Recipients to be notified on job status | |
Attachments | Attachments if any |
Step 3.3. Setup dbt debug job
Field Name | Value | Comment |
Name | job-dbt-debug | |
Script | scripts/job-dbt-debug.py | This is the script that would be executed in this job step. |
Arguments | /home/cdsw/dbt-impala-example/dbt_impala_demo Other command line arguments to dbt | Path of the dbt project file, which is part of the repo. Ex: –profiles-dir |
Editor | Workbench | |
Kernel | Python 3.9 | |
Edition | dbt custom runtime | |
Version | 1.1 | |
Runtime Image | Find most updated docker image here | |
Schedulable | Dependent | Make sure that this job runs only after cloning/updating the git repo. |
job-git-clone | Job-dbt-debug is dependent on job-git-clone and will run only after it completes. | |
Resource profile | 1vCPU/2GiB | |
Timeout In Minutes | - | Optional timeout for the job |
Environment Variables | These can be used to overwrite settings passed at the project level (Section 3.2) | |
Job Report Recipients | Recipients to be notified on job status | |
Attachments | Attachments if any |
Step 3.4. Setup dbt run job
Field Name | Value | Comment |
Name | job-dbt-run | |
Script | scripts/job-dbt-run.py | This is the script which would be executed in this job step. |
Arguments | /home/cdsw/dbt-impala-example/dbt_impala_demo Other command line arguments to dbt | Path of the dbt project file, which is part of the repo. Ex: –profiles-dir |
Editor | Workbench | |
Kernel | Python 3.9 | |
Edition | dbt custom runtime | |
Version | 1.1 | |
Runtime Image | Find most updated docker image here | |
Schedulable | Dependent | Make sure that this job depends on dbt-debug job. |
job-dbt-debug | Job-dbt-run is dependent on job-dbt-debug, and will run only after it completes. | |
Resource profile | 1vCPU/2GiB | |
Timeout In Minutes | - | Optional timeout for the job |
Environment Variables | These can be used to overwrite settings passed at project level (Section 3.2) | |
Job Report Recipients | Recipients to be notified on job status | |
Attachments | Attachments if any |
Step 3.5. Setup dbt docs generate job
Field Name | Value | Comment |
Name | dbt-docs-generate | |
Script | scripts/dbt-docs-generate.py | This is the script which would be executed in this job step. |
Arguments | /home/cdsw/dbt-impala-example/dbt_impala_demo | Path of the dbt project file, which is part of the repo. |
Editor | Workbench | |
Kernel | Python 3.9 | |
Edition | dbt custom runtime | |
Version | 1.1 | |
Runtime Image | Find most updated docker image here | |
Schedulable | Dependent | Generate docs only after the models have been updated. |
dbt-docs-generate | dbt-docs-generate is dependent on job-dbt-run, and will run only after it completes. | |
Resource profile | 1vCPU/2GiB | |
Timeout In Minutes | - | Optional timeout for the job |
Environment Variables | These can be used to overwrite settings passed at project level (Section 3.2) | |
Job Report Recipients | Recipients to be notified on job status | |
Attachments | Attachments if any |
After following the 4 steps above, there will be a pipeline with the 4 jobs that run one after the other, only when the previous job succeeds
Step 4. Create an app to serve documentation
The dbt docs generate job generates static HTML documentation for all the dbt models. In this step, you will create an app to serve the documentation. The script for the app will be available in the custom runtime that is provided.
- Within the Project page, click on Applications
- Create a new Application
- Click Set Environment Variable
Add the environment variable TARGET_PATH. This should be the same path where dbt docs generated the target folder inside the dbt project.Field
Value
Comment
Name
dbt-prod-docs-serve
Domain
dbtproddocs
Script
scripts/dbt-docs-serve.py
Python script to serve the static HTML doc page generated by dbt docs generate. This is part of the CDSW runtime image.
Runtime
dbt custom runtime
dbt custom runtime which was added to the runtime catalog.
Environment Variables
TARGET_PATH
Target folder path for dbt docs.
E.g. /home/cdsw/jaffle_shop/target/
Make sure of the exact path, especially the ‘/’ characters.
Note: To update any of the above parameters go back to application -> Application details. Settings -> update application. Click Restart to restart the application.
A similar application can be set up to extract the dbt logs
Field | Value | Comment |
Name | dbt-logs-serve | |
Domain | dbtlogsserve | |
Script | scripts/dbt-logs-serve.py | Python script to serve the static HTML doc page generated by dbt docs generate. This is part of the CDSW runtime image. |
Runtime | dbt custom runtime | dbt custom runtime which was added to the runtime catalog. |
Environment Variables | TARGET_PATH | Target folder path for dbt docs. E.g. /home/cdsw/jaffle_shop/target/ Make sure of the exact path, especially the ‘/’ characters. |
Description of Production/Stage Deployments
Details and logs for jobs
Logs are available in the workspace in the project folder
The job run details and job logs can be found as follows:
Individual job history can be seen at
Job run details can be seen by selecting one of the runs
Details and logs for doc serve app
Logs for running application can be found in applications->logs
To fetch the logs, launching the application will enable the log file to be downloaded via browser.
Analyst steps
Prerequisites
- Each analyst should have their own credentials to the underlying warehouse. They would need to set a workload password by following Setting the workload password
- Each analyst has their own schema/database to do their development and testing
- Each analyst has access to the git repo with the dbt models and has the ability to create PRs in that git repo with their changes. Admins may have to set up proxies to enable this. If you are creating your own repo as an analyst, refer to this Getting started with dbt Core
- The user SSH key can be configured for access to the git repo (How to work with Github repositories in CML/CDSW - Cloudera Community - 303205)
- Each analyst has access to the custom runtime that is provided by Cloudera. Admins may have to set up proxies to enable this.
- Each analyst has permission to create their own project. We suggest that each analyst create their own dev project to work in isolation from other analysts. If not, Admins will have to create the projects using the steps below and provide access to analysts.
Step 1. Setup a dev project
Step 1.1. Create a CDSW project
- From the workspace screen, click on Add Project
- Fill out the basic information for the project
Field
Value
Notes
Project Name
username-marketing
If not shared project, we suggest prefixing the name of the project with the user name so that it is easily identified
Project Description
Project Visibility
Private
Recommend private for prod
Initial Setup
Blank
- Click the Create Project button on the bottom right corner of the screen
Step 1.2. Set environment variables
To avoid checking profile parameters (user’s credentials) to git, we leverage environment variables that are set at a Project-level.
- Click Project Settings from the side menu on the project home page and click on the Advanced tab
- Enter the environment variables. Click on
to add more environment variables.
Key
Value
Notes
DBT_USER
analyst-user-name
Username used by the analyst. See prerequisites.
DBT_PASSWORD
workload-password
Set the workload password by following Setting the workload password
DBT_HOST
Instance host name
DBT_DBNAME
Db name to be worked on
DBT_SCHEMA
Schema used
Note:
There could be different environment variables that need to be set depending on the specific engine and access methods like Kerberos or LDAP. Refer to the engine-specific adapter documentation to get the full list of parameters in the credentials. - Environment variables will look like as shown below:
- Click the Submit button on the right side of the section
Note:
jaffle_shop: |
Note: Environment variables are really flexible. You can use them for any field in the profiles.yml jaffle_shop: |
Step 2. Setup session for development flow
Step 2.1. Create a new session
- On the project, page click on New Session
- Fill in the form for the session
Field
Value
Notes
Session name
dev-user-session
This private session will be used by the analyst for their work
Runtime
Editor
Workbench
Kernel
Python 3.9
Edition
dbt cdsw custom runtime
Version
1.1
Automatically picked up from the runtime
Enable Spark
Disabled
Runtime image
Automatically picked up
Resource Profile
1 vCPU/2GB Memory
- Click on “Start Session”.
- Click on Terminal Access to open a shell
Step 2.2. Clone dbt repository to start working on it
Clone the repository from within the terminal. Note that the ssh key for git access is a prerequisite.
git clone git@github.com:cloudera/dbt-hive-example.git
Once you clone the repo, you can browse the files in the repo and edit them in the built-in editor.
If the repository does not already have a profiles.yml, create your own yml file within the terminal and run dbt debug to verify that the connection works.
$ mkdir $HOME/.dbt $ cat > $HOME/.dbt/profiles.yml $ cd dbt-impala-example/dbt_impala_demo/ $ dbt debug |
Now you are all set!
You can start making changes to your models in the code editor and testing them.
Conclusion
In this document we have shown the different requirements that need to be met to support the full software development life cycle of dbt models. The table below shows how those requirements have been met.
Requirement | Will this option satisfy the requirement? If yes, how? |
Have multiple environments
| Yes, as explained above. |
Have a dev setup where different users can do the following (in an isolated way): 1. Make changes to models | Yes, per user in their Session in the workspace, having checked out their own branch of the given dbt project codebase. |
2. Test changes | Yes |
3. See logs of tests | Yes |
4. Update docs in the models and see docs | Yes, by running the dbt docs server as a CDSW Application. |
Have a CI/CD pipeline to push committed changes in the git repo to stage/prod environments | Yes, either:
|
See logs in stage/prod of the dbt runs | Yes |
See dbt docs in stage/prod | Yes |
Convenient for analysts - no terminal/shells/installing software on a laptop. Should be able to use a browser. | Yes, he gets a shell via CDSW |
Support isolation across different users using it in dev | Yes, each Session workspace is isolated. |
Support isolation between different environments (dev/stage/prod) | Yes |
Secure login - SAML etc | Yes, controlled by the customer via CDSW |
Be able to upgrade the adapters or core seamlessly | Cloudera will publish new Runtimes. versions of the python packages to PyPI |
Vulnerability scans and fixing CVEs | Cloudera will do scans of the adapters and publish new versions with fixes. |
Ability to run dbt run regularly to update the models in the warehouse | Yes, via CDSW Jobs. |
You can reach out to innovation-feedback@cloudera.com if you have any questions.