Cloudera fast forward are pleased to share our two latest applied machine learning research reports, on Meta-Learning and Structural Time Series. Read on here for more on each report, check-out our blog post to read about our evolving research process, or head on over to our Research Roundup to view our latest research webinar on demand!
Meta-Learning
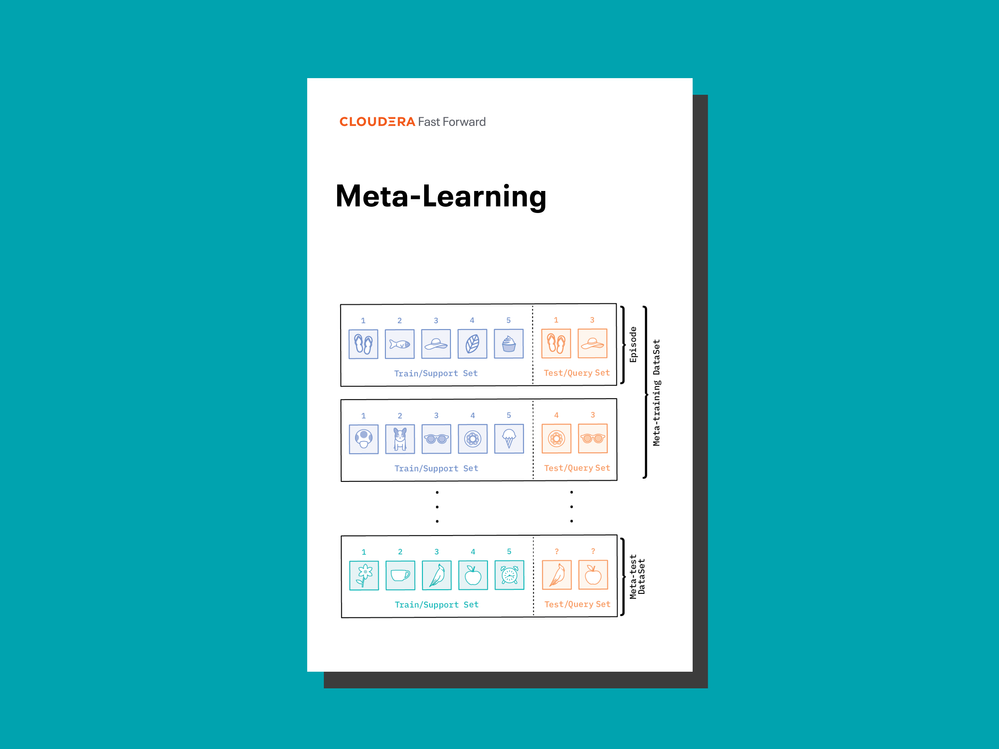
In contrast to how humans learn, deep learning algorithms need vast amounts of data and compute and may yet struggle to generalize. Humans are successful in adapting quickly because they leverage their knowledge acquired from prior experience when faced with new problems. In this webinar we will explain how meta-learning can leverage previous knowledge acquired from data to solve novel tasks quickly and more efficiently during test time.
Our report, Meta-Learning is freely available online, and accompanied by code that applies the technique to an image dataset. Read the report to learn:
- when you should think about meta-learning and lessons to apply in your data science practice
- how meta-learning helps models to generalize to new circumstances or classes during inference
- a foundational approach to the kind of problems it can help us solve, along with our experimental results
Structural Time Series
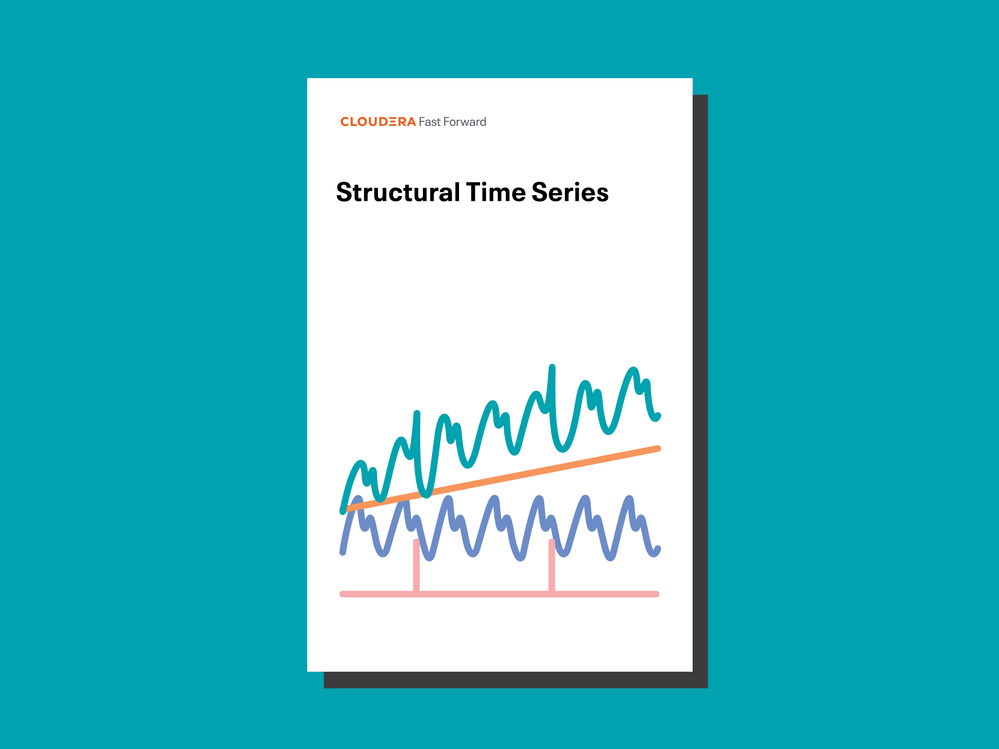
Time series data is ubiquitous, and forecasting has a long history. Generalized additive models give us a simple, flexible and interpretable means for modeling some kinds of time series, especially where there is seasonality. We look at the benefits and trade-offs of taking a curve-fitting approach to time series, and demonstrate its use via Facebook’s Prophet library on a demand forecasting problem.
Our report, Structural Time Series, is freely available online, and accompanied by code applying the techniques discussed to forecasting electricity demand in California. Read the report to learn:
- how capturing the uncertainty in time series allows us to ask better questions
- the importance of baseline models, and how to develop models iteratively
- the trade-offs of a curve-fitting approach to time series